A review of the history of women in computing and AI reveals long-standing barriers to full inclusion and advancement. Computer programming and development was originally considered “women’s work” when the field emerged in the 1800s and early 1900s. For example, the first algorithm meant to be processed by a machine was written by Ada Lovelace in 1843, and the first computer programmers in the 1940s were predominantly women. However, as technology advanced and the field became more prestigious, women were pushed out and excluded from career advancement opportunities. Cultural stereotypes questioning women’s mathematical and analytical competency also contributed to making computing a male-dominated area (Ensmenger, 2010).
Artificial intelligence (AI) has become one of the most transformational technologies of the 21st century. The growth and adoption of AI solutions across industries is rapidly accelerating and the trend presents immense opportunities as well as challenges. The proportion of women in computer science fields, including machine learning, has historically been low compared to men. The data from the US Department of Education indicates that the percentage of women earning bachelor’s degrees specifically in computer sciences has decreased over the past decade (Perry, 2022), although there is a slight increase, from 14.8% in 2009 to 18.7% in 2017 (Elsevier, 2018) and this rise suggests a small improvement in the gender balance amongst those pursuing computer science degrees. This long-term underrepresentation and recent small increase contrast with broader trends of growing participation by women in physical and biological sciences degree programs.
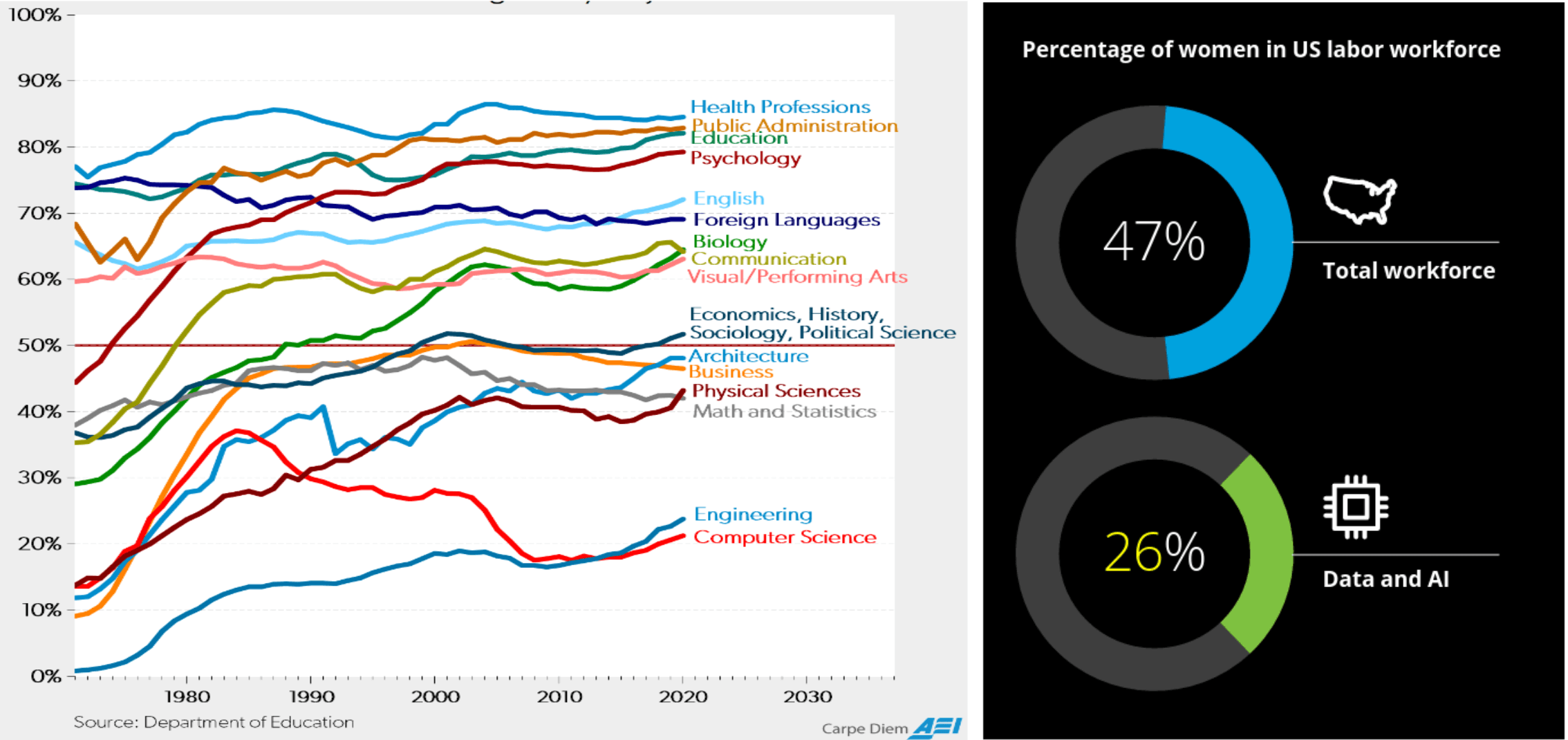
Figure 1: Female Share of Bachelor’s Degrees, 1971 to 2020 and Workforce in the US (Perry, 2022; Deloitte, 2021).
Globally, women are underrepresented in machine learning and data science roles. A 2019 study found that only 12% of machine learning researchers worldwide are female (Kiden et al., 2019). This gender gap persists throughout higher education and the workforce when it comes to roles in data and artificial intelligence. In the United States as of 2020, women made up 47% of the total workforce, and only 26% of those in data and AI roles were women (Deloitte, 2021). This indicates a significant underrepresentation. In sub-Saharan Africa, women have traditionally worked predominantly in agriculture, representing over 50% of the agricultural workforce in the region (Djantchiemo and White, 2022; Masego and Kouakou, 2018). However, there are trends that more African women have been moving into industry and service roles since 2010. Still, their participation in technical fields like artificial intelligence remains low.
According to a 2021 study by Strathmore University, the artificial intelligence (AI) field in Africa suffers from a significant gender imbalance. An analysis of 160 AI companies across 21 African countries found that only 29% of the total AI workforce was female. Moreover, women’s representation in technical roles specifically was even lower in STEM and R&D positions, the representation of female staff ranges between 14-26%. In addition, the study revealed a wider gender gap concentrated at the leadership level in Africa’s AI sector. Women occupied only 10% of the leadership positions and the same percentage represents the female founders of AI startups (Strathmore University, 2021). Furthermore, the analysis of talent across artificial intelligence ecosystems reveals a significant underrepresentation of women, with a study finding only 10% of Google’s AI researchers were female and 25% of IBM’s Africa research team were women (Kiden et al., 2019). This disparity permeates the AI startup ecosystem as well, with just 1 female founder or co-founder out of 21 executives across 14 major companies examined in a report (Kiden et al., 2019). These statistics mirror the global trends of women’s representation in AI.
Figure 2: Gender composition of AI workforce in Africa (Strathmore University, 2021).
Impact of Diversity on Innovation
A report by Lorenzo et al., (2017) showed a correlation between the diverse gender of leadership positions and revenue derived from innovative products and services. Their data show companies where nearly half of managers were women (8 out of 20) realized 34% of revenues from innovations. By contrast, companies with the least gender-diverse managerial cadre (1 out of 20 managers a woman) saw only 25% revenue from innovations (Figure 3).
Figure 3: The proportion of female managers (Lorenzo et al., 2017).
Recent reports highlighted the lack of diversity among AI researchers at major technology companies. Women make up only 15% of AI researchers at Facebook and only 10% at Google (Forbes, 2021; Simonite, 2018). In addition, Black employees make up less than 5% of the workforce at Facebook, Google, and Microsoft, despite representing approximately 12% of the overall US workforce (Microsoft, 2019; Google, 2018; Williams, 2018). Due to the lack of diverse engineers and researchers, the products that are developed and used by billions of users may result in the propagation of bias on a large scale. Hence, inclusion and diversity in AI are crucial.
Challenges
Pipeline Problems
The talent pipeline feeding the AI field is significantly constrained, with women constituting just 22% of AI/machine learning positions and less than 30% of the AI workforce. This underrepresentation stems from social perceptions of computing as a male terrain, dissuading girls from pursuing technology education pathways from a young age (UNESCO, 2019). It limits the diversity of perspectives in AI development.
Bias and Discrimination
Negative stereotypes questioning women’s technical capabilities and societal biases embedded in algorithms contribute to prejudicial evaluations of women’s competencies in AI. These problematic biases also manifest in consumer-facing AI applications like chatbots and virtual assistants, which are often feminized and designed to confirm traditional gender stereotypes regarding women’s societal roles and suitable jobs for women. The datasets and algorithms powering these AI systems frequently fail to represent diverse perspectives and risk perpetuating discrimination through inaccurate or prejudiced interpretations, especially for inputs from certain ethnic or socioeconomic groups. The biases underlying chatbots and assistants mirror the broader challenges women and marginalized communities face regarding equitable participation and treatment in the AI field (European Union, 2020)).
In addition, biases manifest in AI systems as well, producing discriminatory outcomes for women. Algorithmic decisions related to hiring, promotions, salaries and professional advancement opportunities reflect historical employment disparities and gender stereotypes (Buolamwini & Gebru, 2018). This obstructs women’s career progression in AI.
Funding Disparities
Angel investor and venture capital funding data reveals substantial gaps for women AI startup founders, with women receiving less than half as much on average as their male counterparts (Kanze et al., 2018). Such resource constraints pose barriers to succeeding in the AI ecosystem.
Limited Visibility
The low representation of women as AI research authors, speakers and award recipients curtails the visibility of female role models and experts. Women constitute only 13.8% of authorships in AI conference papers and journal articles (Gabriela, 2019). Their perspectives consequently lack prominence in shaping AI priorities and discourse.
Progress
Increasing Representation
The fraction of women seems to be slowly rising among AI students and practitioners, albeit from a low baseline (DeArteaga et al., 2021). Many major AI conferences and journals have also instituted voluntary double-blind review and inclusion initiatives to mitigate bias. For instance, Women in Machine Learning (WiML) supports and empowers women working in machine learning. Participation data from WiML’s annual workshops illustrates rising engagement. In 2010, just 88 people attended WiML’s technical workshop at the Neural Information Processing Systems (NeurIPS) conference. By 2022, attendance swelled over thirteen-fold to 1,157 – showcasing substantial growth in the number of women pursuing machine learning over the past decade. This upward participation trend signals promising advancement and reflects proactive efforts by WiML to cultivate diversity and inclusion in the field through collaborative initiatives like its Un-Workshop event at the International Conference on Machine Learning (ICML). The rising workshop attendance exemplifies positive momentum for women shaping AI’s future. Proactive efforts by companies and universities focused on targeted outreach, family-friendly policies, leadership programs and accountability systems would facilitate improvement.
Legislation.
Several countries have passed mandatory minimum representation legislation for corporate boards and pay transparency regulations to promote equity. Several countries have implemented minimum gender quotas for corporate boards – 40% in Iceland and France, at least 40% in Norway depending on board size, 33% in Italy, and 30% in Germany. While not directly aimed at AI, such measures tackle systemic discrimination issues.
Sustaining Momentum
Maintaining focus on cultivating inclusive cultures and policies remains vital to achieving meaningful progress in addressing multi-faceted gender diversity issues in AI. Anti-harassment systems, grievance redressal channels, bias mitigation practices and equitable funding mechanisms require continued investment and accountability. Expanding access and advancing women from non-traditional backgrounds into education pathways and leadership roles also necessitates persistent efforts across education and industry. Achieving proportionate representation will facilitate shifting discourse and priorities in AI to be more equitable.
References
Buolamwini, J., & Gebru, T. (2018). Gender shades: Intersectional accuracy disparities in commercial gender classification. In Conference on fairness, accountability, and transparency, 77-91. PMLR.
De-Arteaga, M., Romanov, A., Wallach, H., Chayes, J., Borgs, C., Chouldechova, A., Geyik, S., Kenthapadi, K. and Kalai, A.T., 2019, January. Bias in bios: A case study of semantic representation bias in a high-stakes setting. In Proceedings of the Conference on Fairness, Accountability, and Transparency, 120-128.
Deloitte (2021). Women in AI. Available here
Ensmenger, N. (2010). Making programming masculine. Gender codes: Why women are leaving computing, 115-141.
European Union (2020). Gendered Innovations 2: How Inclusive Analysis Contributes to Research and Innovation. Available here
Forbes (2021). Diversity And Inclusion In AI. Available here
Gabriela Ramos (2022).Why we must act now to close the gender gap in AI. Available here
Google. (2018). Google Diversity Annual Report 2018. Available here
Holland, S., Hosny, A., Newman, S., Joseph, J., & Chmielinski, K. (2020). The dataset nutrition label. Data Protection and Privacy, 12(12), 1.
Kanze, D., Huang, L., Conley, M. A., & Higgins, E. T. (2018). We ask men to win and women not to lose: Closing the gender gap in startup funding. Academy of Management Journal, 61(2), 586-614.
Kiden Sarah, Alex Comninos and Chenai Chair (2019). Gender and Artificial Intelligence Readiness in Africa: Can new technological innovations address an unequal playing field? Available here
Lorenzo Rocío, Nicole Voigt, Karin Schetelig, Annika Zawadzki, Isabelle Welpe, and Prisca Brosi (2017). Innovation Through Diversity. Available here
Perry Mark (2022). Animated Chart of the Day: Female Share of US Bachelor’s Degrees, 1971 to 2020. Available here
Masego Madzwamuse and Koffi Kouakou (2018). The future of work for women in Africa: Crises and opportunities. Available here
Microsoft. (2019). Diversity & Inclusion. Available here
Perez, C. C. (2019). Invisible women: Data bias in a world designed for men. Abrams.
Sakinatou Djantchiemo and Tamara White (2022). Figure of the week: Labor trends for women in Africa. Available here
Simonite, T. (2018). AI is the Future—But Where Are the Women? Wired. Available here
Strathmore University (2021). The Artificial Intelligence Labour Gender Gap in Africa. Available here
UNESCO (2019). Gender-responsive STEM education: empowering girls and women for the jobs of today and tomorrow. Available here
West, S. M., Whittaker, M., & Crawford, K. (2019). Discriminating systems. AI Now, 1-33.
Williams, M. (2018). Facebook 2018 Diversity Report: Reflecting on Our Journey. Available here